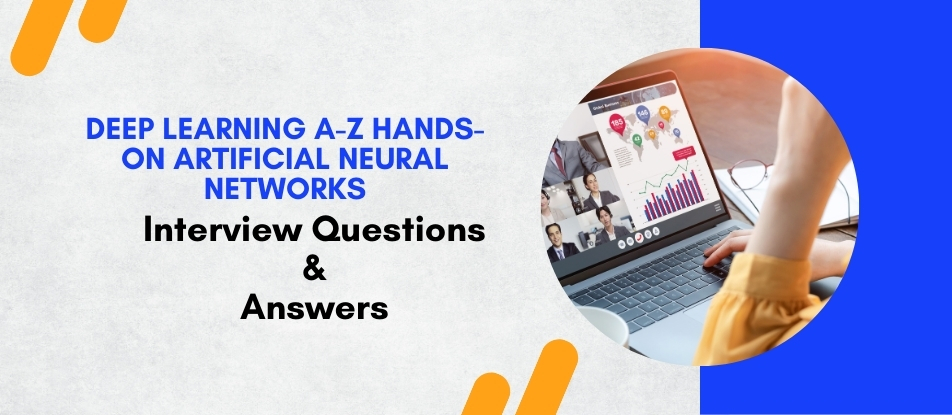
Unlock the power of artificial intelligence with "Deep Learning A-Z Hands-On Artificial Neural Networks Training." This comprehensive 60-hour course covers fundamental to advanced deep learning concepts, including neural networks, CNNs, RNNs, and more. Engage in practical, hands-on projects using Python and TensorFlow to solve real-world problems. Perfect for aspiring data scientists and AI enthusiasts seeking in-depth, actionable knowledge.
Deep Learning A-Z Hands-On Artificial Neural Networks Interview Questions Answers - For Intermediate
1. What is the purpose of activation functions in neural networks?
Activation functions introduce non-linearity into the network, enabling it to learn and model complex patterns. Without them, the network would behave like a linear regression model, limiting its ability to solve intricate tasks such as image and speech recognition.
2. Explain the vanishing gradient problem and its impact on training deep networks.
The vanishing gradient problem occurs when gradients become too small during backpropagation, hindering weight updates in early layers. This slows or stops the training of deep networks, making it difficult for them to learn effectively. Techniques like ReLU activation and residual connections help mitigate this issue.
3. Describe the difference between batch gradient descent and stochastic gradient descent.
Batch gradient descent computes gradients using the entire dataset, ensuring stable convergence but being computationally intensive. Stochastic gradient descent (SGD) updates weights using one sample at a time, offering faster iterations and the ability to escape local minima, though with noisier convergence.
4. What are convolutional neural networks (CNNs) primarily used for, and why?
CNNs are primarily used for image and video recognition tasks. Their convolutional layers effectively capture spatial hierarchies and local patterns through filters, making them adept at recognizing features like edges, textures, and objects within visual data.
5. Explain the role of pooling layers in CNNs.
Pooling layers reduce the spatial dimensions of feature maps, decreasing computational load and controlling overfitting. They summarize the presence of features in regions, typically using operations like max pooling or average pooling, thereby making the network more robust to spatial variations.
6. What is backpropagation and how does it work in neural networks?
Backpropagation is the algorithm for training neural networks by minimizing the loss function. It involves computing the gradient of the loss with respect to each weight using the chain rule and then updating the weights in the opposite direction of the gradient to reduce the error.
7. Define overfitting in the context of neural networks and how to prevent it.
Overfitting occurs when a neural network learns the training data too well, including noise, leading to poor generalization of new data. Techniques to prevent it include regularization (e.g., L2), dropout, early stopping, and using more training data.
8. What is dropout and how does it help in training neural networks?
Dropout is a regularization technique where randomly selected neurons are ignored during training. This prevents units from co-adapting, reduces overfitting, and encourages the network to develop redundant representations, enhancing generalization.
9. Explain the concept of weight initialization and its importance in neural networks.
Proper weight initialization sets initial weights to appropriate values to ensure effective training. Poor initialization can lead to vanishing or exploding gradients, hindering convergence. Techniques like Xavier or He initialization help maintain signal flow and stabilize learning.
10. What are recurrent neural networks (RNNs) and what types of problems are they suited for?
RNNs are neural networks with connections that form directed cycles, enabling them to maintain a hidden state. They are suited for sequential data problems like language modeling, time series prediction, and speech recognition, where context and order are important.
11. Describe the Long Short-Term Memory (LSTM) architecture and its advantages over standard RNNs.
LSTMs are a type of RNN designed to capture long-term dependencies by using gates (input, forget, output) to regulate information flow. They mitigate the vanishing gradient problem, allowing them to remember information over longer sequences compared to standard RNNs.
12. What is a loss function and why is it critical in training neural networks?
A loss function quantifies the difference between the network's predictions and the actual targets. It guides the optimization process by providing a measure to minimize. Choosing an appropriate loss function is crucial for effective learning and achieving desired performance.
13. Explain the concept of learning rate and its effect on neural network training.
The learning rate determines the step size during weight updates. A high learning rate can speed up training but may cause overshooting minima, while a low rate ensures stable convergence but can make training slow. Proper tuning is essential for efficient and effective learning.
14. What are batch normalization layers and how do they improve training?
Batch normalization normalizes the inputs of each layer to have a mean of zero and a variance of one within a mini-batch. This stabilizes and accelerates training by reducing internal covariate shifts, allowing for higher learning rates and reducing sensitivity to initialization.
15. Describe the difference between a fully connected layer and a convolutional layer in neural networks.
A fully connected layer connects every neuron to all neurons in the previous layer, capturing global patterns. In contrast, a convolutional layer applies local filters to input regions, efficiently detecting spatial hierarchies and reducing the number of parameters.
16. What is transfer learning and how is it utilized in deep learning projects?
Transfer learning involves leveraging a pre-trained model on a new, related task. Using the learned features from large datasets, it reduces training time and improves performance, especially when limited data is available for the target task.
17. Explain the concept of gradient clipping and its benefits in training neural networks.
Gradient clipping limits the magnitude of gradients during backpropagation to prevent exploding gradients. This ensures stable and controlled updates, particularly in deep or recurrent networks, facilitating smoother and more reliable training.
18. What is the purpose of an embedding layer in neural networks?
An embedding layer maps discrete inputs, like words, into continuous vector representations. These embeddings capture semantic relationships and reduce dimensionality, enhancing the network's ability to process and understand categorical data effectively.
19. Describe the role of the softmax function in classification tasks.
The softmax function converts raw output scores (logits) into probabilities that sum to one across classes. It is typically used in the output layer for multi-class classification, enabling the network to predict the likelihood of each class.
20. What are attention mechanisms and how do they enhance neural network performance?
Attention mechanisms allow neural networks to focus on specific parts of the input when making predictions. By weighting relevant information more heavily, they improve performance in tasks like machine translation and image captioning, enabling better handling of long-range dependencies.
Deep Learning A-Z Hands-On Artificial Neural Networks Interview Questions Answers - For Advanced
1. Explain the vanishing gradient problem in deep neural networks and how techniques like ReLU and Batch Normalization address it.
The vanishing gradient problem occurs when gradients become too small, hindering weight updates in deep networks. ReLU activation mitigates this by allowing gradients to pass through for positive inputs. Batch Normalization normalizes layer inputs, stabilizing and maintaining gradient magnitudes, which accelerates training and alleviates vanishing gradients
2. Describe the architecture and training process of a Convolutional Neural Network (CNN) used for image classification.
A CNN consists of convolutional layers for feature extraction, pooling layers for dimensionality reduction, and fully connected layers for classification. During training, it uses backpropagation with gradient descent to optimize filters and weights, learning hierarchical feature representations from input images to accurately classify them.
3. What are Generative Adversarial Networks (GANs) and how do the generator and discriminator interact during training?
GANs consist of a generator that creates synthetic data and a discriminator that evaluates authenticity. During training, the generator aims to produce data indistinguishable from real data, while the discriminator strives to correctly classify real versus generated samples. This adversarial process continues until the generator produces highly realistic data.
4. How do Long Short-Term Memory (LSTM) networks address the limitations of traditional RNNs in handling long-term dependencies?
LSTMs incorporate memory cells and gating mechanisms (input, forget, and output gates) that regulate information flow. This structure allows them to maintain and update information over long sequences, effectively capturing long-term dependencies and mitigating issues like vanishing gradients prevalent in traditional RNNs.
5. Explain the concept of transfer learning and its advantages in deep learning applications.
Transfer learning involves leveraging pre-trained models on large datasets and fine-tuning them for specific tasks. Advantages include reduced training time, lower computational resources, improved performance with limited data, and the ability to utilize learned feature representations, making it especially beneficial for tasks with scarce labeled data.
6. What is dropout regularization, and how does it prevent overfitting in neural networks?
Dropout randomly deactivates a subset of neurons during training, forcing the network to learn redundant representations. This prevents reliance on specific neurons, promotes generalization, and reduces overfitting by ensuring the model remains robust and performs well on unseen data.
7. Describe the role of activation functions in neural networks and compare Sigmoid, Tanh, and ReLU in terms of their properties and use cases.
Activation functions introduce non-linearity, enabling networks to learn complex patterns. Sigmoid outputs values between 0 and 1 but suffers from vanishing gradients. Tanh outputs between -1 and 1, offering zero-centered data but similar gradient issues. ReLU is computationally efficient, mitigates vanishing gradients, and is widely used in hidden layers for its simplicity and effectiveness.
8. How does the Adam optimizer improve upon traditional stochastic gradient descent, and what are its key hyperparameters?
Adam combines momentum and adaptive learning rates, maintaining running averages of gradients and squared gradients. This leads to faster convergence and better performance. Key hyperparameters include learning rate (α), β₁ (decay rate for the first moment), β₂ (decay rate for the second moment), and ε (a small constant to prevent division by zero).
9. What are attention mechanisms in neural networks, and how have they revolutionized natural language processing tasks?
Attention mechanisms allow models to focus on specific parts of the input when generating each output element. They enhance the ability to capture dependencies and context, leading to significant improvements in NLP tasks like translation, summarization, and question-answering by enabling more flexible and effective information processing.
10. Explain the concept of convolutional kernel initialization and its impact on training deep neural networks.
Convolutional kernel initialization involves setting initial weights before training. Proper initialization (e.g., He or Xavier) ensures that gradients flow efficiently, preventing issues like vanishing or exploding gradients. It facilitates faster convergence, stable training, and better performance by providing a suitable starting point for weight optimization.
Course Schedule
Mar, 2025 | Weekdays | Mon-Fri | Enquire Now |
Weekend | Sat-Sun | Enquire Now | |
May, 2025 | Weekdays | Mon-Fri | Enquire Now |
Weekend | Sat-Sun | Enquire Now |
Related Courses
Related Articles
- Learn Microsoft Azure Fundamentals (AZ-900) with Best Azure Course Online
- Transform Your Skills with Palantir Foundry Data Science Course
- Sharpen Your Skills: Become Certified in Caesar II Pipe Stress Analysis
- How Salesforce Health Cloud is Transforming Healthcare CRM?
- How Salesforce Financial Services Cloud is Redefining Wealth Management?
Related Interview
Related FAQ's
- Instructor-led Live Online Interactive Training
- Project Based Customized Learning
- Fast Track Training Program
- Self-paced learning
- In one-on-one training, you have the flexibility to choose the days, timings, and duration according to your preferences.
- We create a personalized training calendar based on your chosen schedule.
- Complete Live Online Interactive Training of the Course
- After Training Recorded Videos
- Session-wise Learning Material and notes for lifetime
- Practical & Assignments exercises
- Global Course Completion Certificate
- 24x7 after Training Support
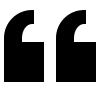