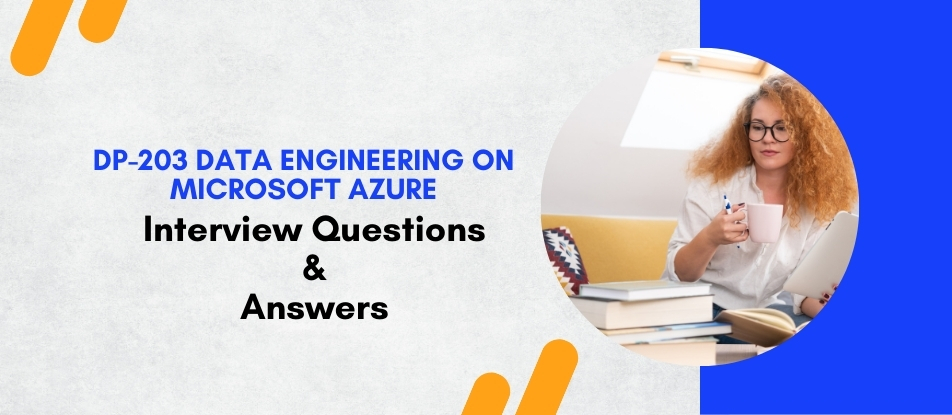
The DP-203: Data Engineering on Microsoft Azure training course is designed for professionals looking to master data engineering principles using Microsoft Azure's data services. Participants will learn to design and implement data storage solutions, manage and develop data processing, and monitor and optimize data solutions. The course covers Azure Synapse Analytics, Azure Data Lake Storage, Azure Data Factory, and Azure Stream Analytics, preparing candidates for the Microsoft Certified: Azure Data Engineer Associate exam.
DP-203 Data Engineering on Microsoft Azure Interview Questions Answers - For Intermediate
1. What is Azure Data Factory (ADF)?
ADF is a cloud-based data integration service that allows you to create, schedule, and manage data pipelines for data movement and transformation.
2. How do you move data from on-premises to Azure using Azure Data Factory?
We can use the Copy Data activity in ADF to move data from on-premises to Azure. This involves creating linked services for both the source and destination data stores and configuring the Copy Data activity to specify the data movement.
3. What is a linked service in Azure Data Factory?
A linked service is a configuration object that defines the connection information for a data store or a compute resource in Azure Data Factory.
4. Explain the difference between a pipeline and a dataset in Azure Data Factory.
A pipeline is a logical grouping of activities that together perform a task, such as moving data from source to destination. A dataset defines the schema and location of the data used as input or output by activities within a pipeline.
5. What are the different types of activities in Azure Data Factory?
Activities in Azure Data Factory include data movement activities (e.g., Copy Data), data transformation activities (e.g., Data Flow), control activities (e.g., ForEach), and data flow activities (e.g., Lookup).
6. How do you monitor and manage Azure Data Factory pipelines?
Azure Data Factory provides monitoring capabilities through Azure Monitor and Azure Data Factory Monitor. We can use these tools to monitor pipeline runs, trigger pipelines, and manage alerts.
7. What is a Data Flow in Azure Data Factory?
Data Flow is a cloud-based data integration service that allows you to visually design and execute data transformations at scale within Azure Data Factory.
8. How do you parameterize Azure Data Factory pipelines?
We can parameterize pipelines in Azure Data Factory by defining parameters at the pipeline level and passing values dynamically during runtime using parameterized expressions.
9. What is Azure Data Lake Storage (ADLS)?
Azure Data Lake Storage is a scalable and secure cloud-based storage solution optimized for big data analytics workloads. It enables you to store and analyze structured and unstructured data of any size.
10. How can you schedule data integration tasks in Azure Data Factory?
Data integration tasks in Azure Data Factory can be scheduled using triggers. Triggers can be time-based (e.g., schedule trigger) or event-based (e.g., tumbling window trigger) to automate pipeline execution based on specific criteria.
11. What is Azure Synapse Analytics?
Azure Synapse Analytics is an analytics service that combines enterprise data warehousing and big data analytics. It provides capabilities for data integration, data warehousing, big data analytics, and machine learning.
12. How do you ingest streaming data into Azure Data Lake Storage using Azure Data Factory?
Streaming data can be ingested into Azure Data Lake Storage using Azure Data Factory by configuring a streaming data source as the source dataset and specifying the sink dataset as Azure Data Lake Storage. Then, you can use the Copy Data activity with a streaming dataset as the source.
13. Explain the difference between Azure Blob Storage and Azure Data Lake Storage.
Azure Blob Storage is a general-purpose object storage solution for storing unstructured data, while Azure Data Lake Storage is optimized for big data analytics workloads and provides capabilities for storing both structured and unstructured data in a hierarchical namespace.
14. What is PolyBase in Azure Synapse Analytics?
PolyBase is a feature in Azure Synapse Analytics that enables you to query and analyze data across relational databases and big data stores using standard T-SQL queries.
15. How do you perform data transformation using Azure Databricks in Azure Data Factory?
Data transformation using Azure Databricks in Azure Data Factory involves creating a Databricks notebook with the necessary transformation logic and executing it using the Azure Databricks activity within a Data Factory pipeline.
16. What is a Data Lake in Azure?
A Data Lake in Azure is a centralized repository that allows you to store structured and unstructured data at any scale. It provides capabilities for data storage, data analytics, and data processing.
17. How do you implement incremental data loading in Azure Data Factory?
Incremental data loading in Azure Data Factory can be implemented by using watermark columns or change tracking mechanisms to identify new or updated data since the last data load and only load the incremental changes.
18. What is the difference between Azure SQL Database and Azure SQL Data Warehouse?
Azure SQL Database is a fully managed relational database service optimized for OLTP workloads, while Azure SQL Data Warehouse is a fully managed data warehousing service optimized for analytics and reporting workloads.
19. How do you handle schema evolution in Azure Data Lake Storage?
Schema evolution in Azure Data Lake Storage can be handled by using schema-on-read techniques, where the schema is applied at the time of data access, allowing for flexible data ingestion and querying.
20. What are the security features available in Azure Data Factory?
Azure Data Factory provides security features such as encryption at rest and in transit, role-based access control (RBAC), managed identities, and integration with Azure Key Vault for securely storing and managing credentials and secrets.
DP-203 Data Engineering on Microsoft Azure Interview Questions Answers - For Advanced
1. How would you optimize data partitioning in Azure Synapse Analytics to improve query performance?
In Azure Synapse Analytics, data partitioning is crucial for enhancing query performance, especially for large datasets. To optimize, one should first understand the data access patterns and partition the data accordingly. For example, partitioning data by date or business unit can significantly reduce the amount of data scanned during queries. Using column store indexes on partitioned tables can also improve performance, as they compress data and reduce I/O.
2. Explain how you can use Azure Data Factory to handle schema drift in data flows.
Azure Data Factory can manage schema drift using its Schema Drift feature in data flows, which allows pipelines to automatically adapt to changes in the source data schema. This is achieved by enabling the 'Allow schema drift' option in the source transformation settings, and using the 'Derived Column' or 'Select' transformations to dynamically manage changes in the schema, such as adding, removing, or transforming columns as needed.
3. Discuss the integration of Azure Stream Analytics with IoT devices for real-time analytics.
Azure Stream Analytics seamlessly integrates with IoT devices through IoT Hubs or Event Hubs, providing a real-time analytics solution that can process large streams of data from various devices. This integration enables the analysis of data in motion, which is crucial for scenarios like monitoring environmental conditions or equipment health in real time. Stream Analytics supports complex event processing, and temporal analytics, and can output data to databases, files, or dashboards for further analysis.
4. What strategies would you use to secure data in Azure Data Lake Storage Gen2?
To secure data in Azure Data Lake Storage Gen2, a combination of access control, network security, and encryption should be employed. Access control can be managed through Azure Active Directory and role-based access control (RBAC) to ensure that only authorized users can access data. Network security can be enhanced by enabling firewalls and virtual network integration. Finally, data should be encrypted at rest using Azure-managed keys or customer-managed keys and during transit using SSL/TLS.
5. How do you ensure data quality when using Azure Data Factory for data integration?
Ensuring data quality in Azure Data Factory involves several practices. Firstly, use the data flow's 'Data Flow Debug' feature to preview data and validate transformations. Implementing data validation rules, such as checking for null values or incorrect formats, can help maintain quality. Additionally, leveraging Azure Monitor to track pipeline runs and data integration outcomes helps identify and rectify issues promptly.
6. Can you explain the use of PolyBase for data loading into Azure SQL Data Warehouse (Synapse Analytics), and its advantages?
PolyBase is a technology that enables SQL queries to perform T-SQL queries directly against external data stored in Hadoop or Azure Blob Storage. It is used in Azure Synapse Analytics for efficient data loading as it allows using T-SQL statements to import data directly into SQL Data Warehouse. The advantages include the ability to handle large volumes of data without moving the data into a separate staging area, reducing ETL time and resources.
7. What is the role of Azure Databricks in the Azure data ecosystem, and how does it integrate with other Azure services?
Azure Databricks is an Apache Spark-based analytics platform optimized for the Microsoft Azure cloud services platform. It plays a critical role in the Azure data ecosystem by providing a high-performance engine for big data processing and machine learning. Databricks integrates with Azure services like Azure Data Lake Storage, Azure SQL Data Warehouse, and Azure Cosmos DB, enabling a seamless data processing pipeline that supports both batch and real-time processing.
8. Describe how you would implement disaster recovery for Azure SQL databases.
For disaster recovery in Azure SQL databases, it is essential to implement a strategy that includes automated backups, geo-replication, and failover groups. Automated backups should be configured according to the recovery point objective (RPO). Geo-replication enables the replication of data to a secondary region, and failover groups can be used to manage the failover of databases to the secondary location automatically in the event of a disaster.
9. How do you monitor and optimize Azure Data Factory pipelines?
Monitoring and optimizing Azure Data Factory pipelines involves using Azure Monitor and Azure Data Factory's monitoring features. Pipelines should be designed with logging in mind, capturing details of each activity run. Performance issues can often be mitigated by optimizing the design of the pipelines, such as adjusting parallelism, tuning the performance of the underlying data stores, and redesigning components for better efficiency.
10. Explain the concept of time windowing in Azure Stream Analytics and its applications.
Time windowing in Azure Stream Analytics refers to the process of aggregating data over a set period, which is essential for handling streams of data in real time. This technique is used to perform calculations across a window of data, such as summing up sales every minute. Applications of time windowing include real-time monitoring, event detection, and temporal analytics, crucial for scenarios that require timely insights from streaming data.
Course Schedule
Feb, 2025 | Weekdays | Mon-Fri | Enquire Now |
Weekend | Sat-Sun | Enquire Now | |
Mar, 2025 | Weekdays | Mon-Fri | Enquire Now |
Weekend | Sat-Sun | Enquire Now |
Related Courses
Related Articles
Related Interview
Related FAQ's
- Instructor-led Live Online Interactive Training
- Project Based Customized Learning
- Fast Track Training Program
- Self-paced learning
- In one-on-one training, you have the flexibility to choose the days, timings, and duration according to your preferences.
- We create a personalized training calendar based on your chosen schedule.
- Complete Live Online Interactive Training of the Course
- After Training Recorded Videos
- Session-wise Learning Material and notes for lifetime
- Practical & Assignments exercises
- Global Course Completion Certificate
- 24x7 after Training Support
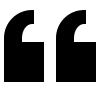