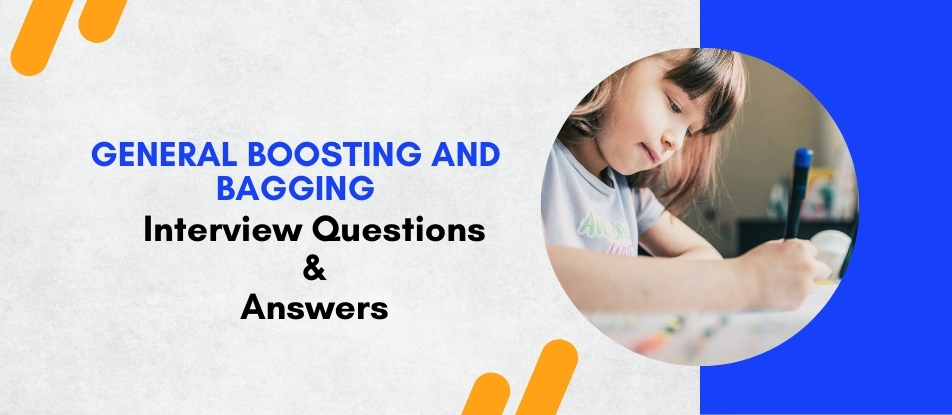
Enhance your machine learning skills with our General Boosting and Bagging Online Training. Dive into ensemble techniques, understand core concepts, and apply practical strategies to improve model accuracy and robustness. Through interactive lessons, real-world examples, and expert guidance, you'll master boosting and bagging methods essential for data scientists and analysts. Elevate your expertise and drive impactful results today.
General Boosting and Bagging Interview Questions Answers - For Intermediate
1. What is the primary difference between bagging and boosting in ensemble methods?
Bagging (Bootstrap Aggregating) builds multiple models independently using different subsets of data and aggregates their predictions to reduce variance. Boosting, however, builds models sequentially, where each model focuses on correcting the errors of the previous ones, thereby reducing bias and improving accuracy.
2. How does online bagging differ from traditional bagging?
Online bagging adapts the bagging technique for streaming data by assigning weights to instances probabilistically, allowing incremental model updates. Unlike traditional bagging, which relies on multiple bootstrap samples, online bagging processes data in a single pass without storing the entire dataset.
3. What are the advantages of using boosting in online training?
Boosting online training can continuously improve model accuracy by focusing on misclassified instances. It adapts to evolving data patterns, enhances predictive performance by reducing bias, and efficiently handles large-scale data streams through incremental updates.
4. Explain the concept of adaptive boosting (AdaBoost) in the context of online learning.
In online learning, AdaBoost assigns weights to instances, increasing weights for misclassified samples. As data arrives sequentially, the model updates incrementally, emphasizing difficult cases. This adaptive weighting helps the ensemble focus on errors, improving classification accuracy over time.
5. What challenges arise when implementing bagging for online training?
Challenges include managing memory efficiently since data streams are potentially infinite, ensuring models are updated incrementally without retraining from scratch, handling concept drift, and maintaining diversity among ensemble members to preserve the bagging benefits.
6. How can Random Forests be adapted for online bagging?
Random Forests can be adapted for online bagging by maintaining multiple decision trees that are incrementally updated with incoming data. Each tree receives a weighted subset of the data stream, and predictions are aggregated. Techniques like reservoir sampling can manage memory and ensure diversity among trees.
7. Describe the role of weighting in online boosting algorithms.
Weighting in online boosting assigns higher importance to misclassified instances, guiding subsequent models to focus on these harder cases. This dynamic adjustment helps the ensemble correct errors progressively, enhancing overall model accuracy and adaptability to new data.
8. What is concept drift, and how do boosting and bagging handle it in online training?
Concept drift refers to changes in the underlying data distribution over time. Boosting handles it by continuously updating model weights to adapt to new patterns, while bagging manages it by maintaining diverse models that can respond to shifts, ensuring the ensemble remains robust against evolving data.
9. Compare the computational complexity of boosting and bagging in online settings.
Boosting typically has higher computational complexity due to its sequential model training and weight adjustments. Bagging, being parallelizable with independent models, often has lower complexity per model. However, both must efficiently handle incremental updates to suit online environments.
10. How does ensemble diversity affect the performance of online bagging?
Ensemble diversity ensures that individual models make different errors, enhancing the overall ensemble’s ability to generalize. In online bagging, maintaining diversity through varied data subsets or different model parameters prevents correlated errors, improving accuracy and robustness.
11. What is online Gradient Boosting, and how does it differ from batch Gradient Boosting?
Online Gradient Boosting incrementally updates models with each new data point, adjusting gradients on the fly. Unlike batch Gradient Boosting, which processes the entire dataset in iterations, online Gradient Boosting efficiently handles streaming data and adapts to changes in real time.
12. Explain the concept of streaming ensembles in the context of online bagging and boosting.
Streaming ensembles consist of multiple models that are continuously updated as data streams. In online bagging, models receive weighted instances independently, while in boosting, models sequentially focus on correcting errors. This approach ensures the ensemble adapts to new data and maintains high performance.
13. How can model drift be detected and addressed in online boosting?
Model drift can be detected using monitoring metrics like accuracy over time or error rates. To address it, online boosting can adjust instance weights, retrain or replace outdated models, and incorporate mechanisms to forget or down-weight older data, ensuring the ensemble remains relevant.
14. What role does base learner selection play in online boosting and bagging?
Base learner selection is crucial as it affects the ensemble’s ability to learn and adapt. In online boosting and bagging, simple and fast learners like decision stumps are often preferred for efficiency. The choice impacts the ensemble’s accuracy, computational cost, and adaptability to streaming data.
15. Describe how incremental learning is applied in online bagging.
Incremental learning in online bagging involves updating each base model with new data as it arrives without retraining from scratch. Models receive weighted instances based on probabilistic sampling, allowing the ensemble to grow and adapt continuously while maintaining diversity and accuracy.
16. What is the impact of learning rate in online boosting algorithms?
The learning rate controls the contribution of each new model in boosting. A lower learning rate makes the ensemble update more gradually, enhancing stability and preventing overfitting, while a higher rate allows faster adaptation. Balancing it is essential for optimal performance in online boosting.
17. How can ensemble size be managed in online bagging to ensure efficiency?
Ensemble size in online bagging can be managed by limiting the number of base models, using model pruning strategies, or employing sliding windows to keep only recent models. This ensures computational efficiency, and memory management, and maintains the ensemble’s relevance to current data.
18. What techniques can enhance the robustness of online boosting against noisy data?
Techniques include using regularization to prevent overfitting, incorporating noise detection mechanisms to down-weight or ignore noisy instances, employing robust loss functions, and maintaining diverse base learners to mitigate the impact of noise on the overall ensemble.
19. How does online boosting handle imbalanced data streams?
Online boosting addresses imbalanced data by adjusting instance weights to emphasize minority classes, using cost-sensitive learning, resampling techniques, or integrating specialized base learners that focus on correctly classifying underrepresented classes, thereby improving the ensemble’s performance on imbalanced streams.
20. What are some real-world applications of online boosting and bagging?
Real-world applications include real-time fraud detection, where models must adapt to new fraudulent patterns; online recommendation systems that personalize suggestions based on user behavior; adaptive spam filtering; and dynamic risk assessment in finance, all benefiting from the adaptability and robustness of online boosting and bagging ensembles.
General Boosting and Bagging Interview Questions Answers - For Advanced
1. How do online boosting algorithms handle concept drift in streaming data?
Online boosting algorithms address concept drift by continuously updating weak learners with new data and possibly weighting or replacing outdated models. Techniques like adaptive boosting adjust the weights of instances based on recent performance, ensuring the ensemble remains responsive to changes in data distribution over time.
2. What are the key differences between Bagging and Boosting in an online training context?
In online training, Bagging builds multiple independent models on bootstrap samples of streaming data, promoting diversity and reducing variance. Boosting sequentially trains models, focusing on correcting errors of previous learners by reweighting instances, thereby reducing bias. Bagging emphasizes parallelism while Boosting emphasizes model dependency and adaptability.
3. Explain how Online Bagging can be efficiently implemented with limited memory resources.
Online Bagging can utilize techniques like reservoir sampling to maintain representative subsets of data streams. It employs incremental updates where each incoming instance is probabilistically assigned to multiple models. By sharing models and using lightweight data structures, it minimizes memory usage while ensuring diverse ensemble members for effective generalization.
4. Describe the role of instance weighting in online boosting algorithms.
Instance weighting in online boosting assigns higher weights to misclassified or difficult instances, guiding subsequent weak learners to focus on these areas. This adaptive emphasis helps the ensemble correct previous errors, enhancing overall model accuracy. Proper weight adjustment is crucial for maintaining balance and preventing overfitting in the evolving data stream.
5. How does the Adaptive Boosting (AdaBoost) algorithm extend to online learning scenarios?
Online AdaBoost adapts by updating instance weights incrementally as each data point arrives. It maintains a set of weak learners that are updated or replaced based on performance. The algorithm adjusts the influence of each learner in the ensemble dynamically, ensuring that the model remains robust and responsive to new information in the stream.
6. What challenges arise when applying Bagging to highly imbalanced data streams in an online setting?
Challenges include maintaining minority class representation across ensemble members, preventing bias towards majority classes, and ensuring efficient sampling without overloading memory. Online Bagging must implement strategies like adaptive sampling or cost-sensitive learning to preserve class balance, ensuring that the ensemble effectively learns from imbalanced streams.
7. Compare the computational complexities of online Bagging and Boosting algorithms.
Online Bagging typically has lower computational complexity due to parallel independent model updates, allowing scalability with data streams. Online Boosting involves sequential updates and instance weighting, which can be more computationally intensive. However, optimizations like shared computations and lightweight learners can mitigate Boosting's higher complexity in practice.
8. How can ensemble diversity be maintained in online Bagging to enhance performance?
Diversity in online Bagging is maintained by using different bootstrap samples through probabilistic instance selection, employing varied model architectures, or initializing models with different parameters. Additionally, incorporating random feature selection or leveraging different data subsets ensures that ensemble members make varied predictions, enhancing overall performance.
9. Explain the concept of regret minimization in the context of online Boosting.
Regret minimization in online Boosting involves ensuring that the ensemble's cumulative loss approaches that of the best possible fixed model in hindsight. It measures the difference between the algorithm's performance and the optimal benchmark, guiding the Boosting process to adaptively reduce errors and improve decision-making over time in dynamic environments.
10. What strategies can be employed to prevent overfitting in online Boosting models?
Strategies include limiting the number of weak learners, using regularization techniques, incorporating early stopping based on validation performance, and employing shrinkage (learning rate) to control updates. Additionally, maintaining diversity among learners and using ensemble pruning can help prevent overfitting, ensuring the model generalizes well to new data.
Course Schedule
Mar, 2025 | Weekdays | Mon-Fri | Enquire Now |
Weekend | Sat-Sun | Enquire Now | |
May, 2025 | Weekdays | Mon-Fri | Enquire Now |
Weekend | Sat-Sun | Enquire Now |
Related Courses
Related Articles
Related Interview
Related FAQ's
- Instructor-led Live Online Interactive Training
- Project Based Customized Learning
- Fast Track Training Program
- Self-paced learning
- In one-on-one training, you have the flexibility to choose the days, timings, and duration according to your preferences.
- We create a personalized training calendar based on your chosen schedule.
- Complete Live Online Interactive Training of the Course
- After Training Recorded Videos
- Session-wise Learning Material and notes for lifetime
- Practical & Assignments exercises
- Global Course Completion Certificate
- 24x7 after Training Support
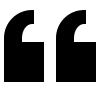