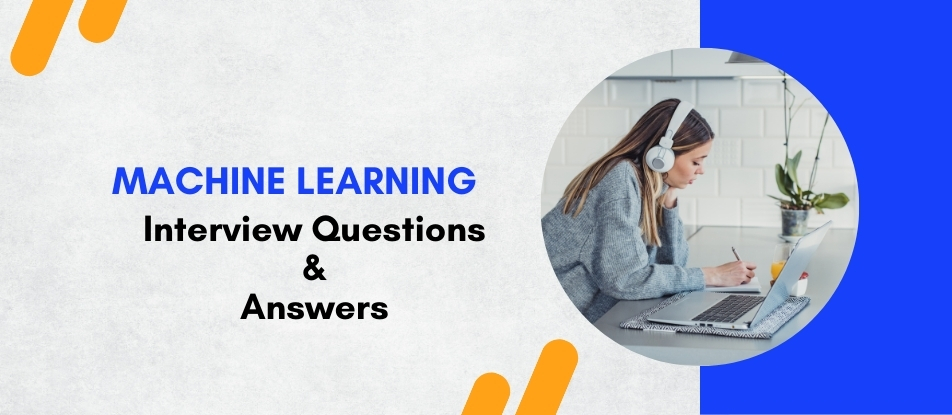
Advance your career with our Machine Learning Online Training and Certification Course. Learn essential algorithms, data processing, and model deployment through engaging, interactive modules and practical projects. Suitable for beginners and professionals, this flexible online program provides expert-led instruction, comprehensive materials, and a recognized certification, empowering you to master machine learning and excel in the AI-driven industry.
Machine Learning Interview Questions Answers - For Intermediate
1. What is the bias-variance tradeoff in machine learning?
The bias-variance tradeoff refers to the balance between a model's ability to minimize bias (error from erroneous assumptions) and variance (error from sensitivity to fluctuations in training data). High bias can cause underfitting, while high variance can lead to overfitting. Optimal performance requires minimizing both to achieve generalization.
2. Explain the difference between supervised and unsupervised learning.
Supervised learning uses labeled data to train models to predict outcomes, such as classification and regression tasks. Unsupervised learning deals with unlabeled data, aiming to find hidden patterns or intrinsic structures, like clustering and dimensionality reduction.
3. What is cross-validation and why is it used?
Cross-validation is a technique for assessing a model’s performance by partitioning data into subsets, training on some and validating on others. It helps in mitigating overfitting, ensuring the model generalizes well to unseen data. Common methods include k-fold and stratified cross-validation.
4. Describe the purpose of regularization in machine learning.
Regularization adds a penalty to the loss function to constrain model complexity, preventing overfitting. Techniques like L1 (Lasso) and L2 (Ridge) regularization shrink coefficient values, promoting simpler models that generalize better to new data.
5. What is a confusion matrix and what metrics can be derived from it?
A confusion matrix is a table summarizing a classification model’s performance, showing true vs. predicted labels. Metrics derived include accuracy, precision, recall, F1-score, and specificity, which help evaluate different aspects of the model’s predictive capabilities.
6. Explain the concept of feature engineering.
Feature engineering involves creating, selecting, or transforming variables to improve model performance. It includes techniques like normalization, encoding categorical variables, creating interaction terms, and extracting relevant features, thereby enhancing the model’s ability to learn patterns.
7. What is the difference between bagging and boosting?
Bagging (Bootstrap Aggregating) builds multiple models independently and aggregates their predictions to reduce variance, e.g., Random Forest. Boosting sequentially builds models, each correcting errors of the previous, thereby reducing bias and improving accuracy, e.g., Gradient Boosting.
8. Define overfitting and underfitting in machine learning models.
Overfitting occurs when a model learns noise and details from training data, performing poorly on new data. Underfitting happens when a model is too simple to capture underlying patterns, leading to poor performance on both training and testing data.
9. What are hyperparameters and how are they different from model parameters?
Hyperparameters are settings configured before training, such as learning rate, number of trees, or regularization strength. Model parameters are learned from data during training, like weights in a neural network. Hyperparameter tuning optimizes model performance.
10. Explain the role of activation functions in neural networks.
Activation functions introduce non-linearity into neural networks, enabling them to learn complex patterns. Common functions include ReLU, sigmoid, and tanh. They determine the output of neurons, influencing how signals propagate through the network.
11. What is Principal Component Analysis (PCA) and its use case?
PCA is a dimensionality reduction technique that transforms data into orthogonal principal components, capturing maximum variance. It reduces feature space, mitigates multicollinearity, and enhances visualization, often used in preprocessing for machine learning models.
12. Describe the k-Nearest Neighbors (k-NN) algorithm.
k-NN is a non-parametric, instance-based learning algorithm used for classification and regression. It predicts the label of a data point based on the majority class among its k closest neighbors in the feature space, using distance metrics like Euclidean.
13. What is the purpose of the learning rate in gradient descent?
The learning rate controls the step size during optimization in gradient descent. A proper learning rate ensures efficient convergence to the minimum loss. Too high can cause overshooting, while too low can result in slow training or getting stuck in local minima.
14. Explain what a support vector machine (SVM) does.
SVM is a supervised learning algorithm for classification and regression. It finds the optimal hyperplane that maximizes the margin between different classes. SVM can handle non-linear boundaries using kernel functions like RBF, polynomial, or sigmoid.
15. What is ensemble learning and its benefits?
Ensemble learning combines multiple models to improve predictive performance. Benefits include enhanced accuracy, robustness, and reduced overfitting. Techniques include bagging, boosting, and stacking, leveraging the strengths of diverse models.
16. Define precision and recall in the context of classification.
Precision is the ratio of true positive predictions to total positive predictions, indicating the accuracy of positive predictions. Recall (sensitivity) is the ratio of true positives to actual positives, measuring the model’s ability to identify all relevant instances.
17. What are the ROC curve and AUC?
The ROC (Receiver Operating Characteristic) curve plots the true positive rate against the false positive rate at various threshold settings. AUC (Area Under the Curve) quantifies overall model performance, with higher values indicating better discrimination between classes.
18. Explain the difference between batch and online learning.
Batch learning trains models on the entire dataset at once, suitable for static data. Online learning updates models incrementally as new data arrives, ideal for dynamic or streaming data scenarios, allowing real-time adaptation.
19. What is a confusion matrix and what metrics can be derived from it?
A confusion matrix is a table summarizing a classification model’s performance, showing true vs. predicted labels. Metrics derived include accuracy, precision, recall, F1-score, and specificity, which help evaluate different aspects of the model’s predictive capabilities.
20. Describe the concept of gradient boosting.
Gradient boosting is an ensemble technique that builds models sequentially, each correcting errors of the previous by optimizing a loss function. It combines weak learners, typically decision trees, to create a strong predictive model, effectively reducing bias and variance.
Machine Learning Interview Questions Answers - For Advanced
1. Explain the Bias-Variance Tradeoff in Machine Learning.
The bias-variance tradeoff balances model simplicity and complexity. High bias leads to underfitting, where the model is too simple to capture data patterns. High variance causes overfitting, where the model captures noise. Optimal performance is achieved by minimizing both bias and variance, ensuring the model generalizes well to unseen data.
2. Describe the Working Principle of Gradient Boosting Machines (GBM).
GBM builds an ensemble of weak learners, typically decision trees, sequentially. Each new tree corrects errors made by the previous ones by focusing on residuals. The final prediction aggregates the contributions of all trees, usually via weighted sums, enhancing accuracy and reducing bias.
3. What are the advantages of using Convolutional Neural Networks (CNNs) for image data?
CNNs effectively capture spatial hierarchies through convolutional layers, reducing parameters via weight sharing. They automatically learn feature representations, handle translation invariance, and are highly scalable, making them ideal for image recognition, classification, and detection tasks.
4. How does Principal Component Analysis (PCA) aid in dimensionality reduction?
PCA transforms data into a new coordinate system, identifying principal components with maximum variance. Selecting top components, it reduces dimensionality while preserving most information, mitigating the curse of dimensionality and enhancing computational efficiency.
5. Explain the concept of Regularization and its types in machine learning.
Regularization prevents overfitting by adding penalty terms to the loss function. Common types include L1 (lasso) promoting sparsity, L2 (ridge) discouraging large weights, and Elastic Net, which combines both. These techniques constrain model complexity, enhancing generalization.
6. What is the role of the activation function in neural networks, and why is non-linearity important?
Activation functions introduce non-linearity, enabling neural networks to model complex relationships. Without non-linear functions, networks would behave like linear models regardless of depth. Common activations include ReLU, sigmoid, and tanh, each aiding in different aspects like gradient flow and output range.
7. Describe the concept of Reinforcement Learning and its key components.
Reinforcement Learning (RL) involves an agent interacting with an environment to maximize cumulative rewards. Key components include states, actions, rewards, policies, and value functions. The agent learns optimal strategies through exploration and exploitation, balancing immediate and future rewards.
8. How does the Expectation-Maximization (EM) algorithm work for parameter estimation?
The EM algorithm iteratively estimates parameters in models with latent variables. The Expectation (E) step computes the expected values of latent variables given current parameters. The Maximization (M) step updates parameters to maximize the likelihood based on these expectations. This continues until convergence.
9. What are Generative Adversarial Networks (GANs) and their primary applications?
GANs consist of a generator and discriminator in a competitive framework. The generator creates fake data, while the discriminator evaluates authenticity. Through adversarial training, GANs learn to produce realistic data. Applications include image synthesis, data augmentation, and style transfer.
10. Explain the difference between Batch Gradient Descent and Stochastic Gradient Descent.
Batch Gradient Descent computes gradients using the entire dataset per update, ensuring stable convergence but being computationally intensive. Stochastic Gradient Descent (SGD) updates parameters using one sample at a time, offering faster, more frequent updates but with noisier convergence, which can help escape local minima.
Course Schedule
Feb, 2025 | Weekdays | Mon-Fri | Enquire Now |
Weekend | Sat-Sun | Enquire Now | |
Mar, 2025 | Weekdays | Mon-Fri | Enquire Now |
Weekend | Sat-Sun | Enquire Now |
Related Courses
Related Articles
Related Interview
Related FAQ's
- Instructor-led Live Online Interactive Training
- Project Based Customized Learning
- Fast Track Training Program
- Self-paced learning
- In one-on-one training, you have the flexibility to choose the days, timings, and duration according to your preferences.
- We create a personalized training calendar based on your chosen schedule.
- Complete Live Online Interactive Training of the Course
- After Training Recorded Videos
- Session-wise Learning Material and notes for lifetime
- Practical & Assignments exercises
- Global Course Completion Certificate
- 24x7 after Training Support
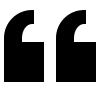