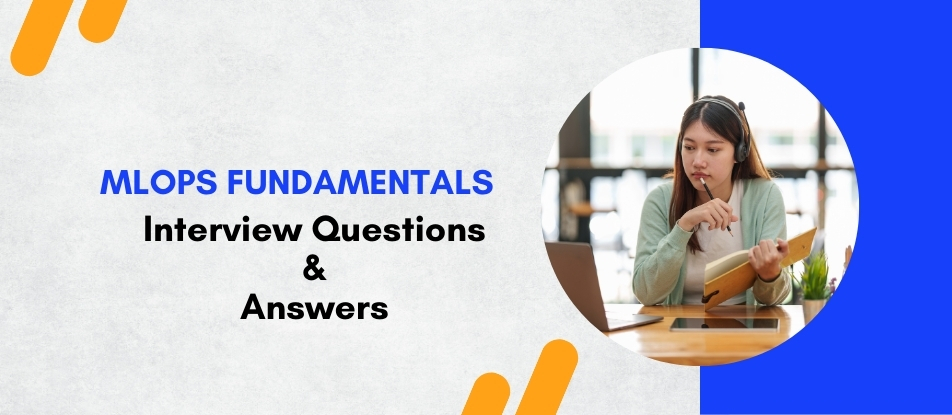
Unlock the power of MLOps with our online Fundamentals Training Course. Learn to integrate machine learning into production seamlessly through CI/CD pipelines, automated deployments, scalability, and monitoring. Engage in hands-on projects using industry tools and best practices to bridge data science and IT operations. Ideal for professionals aiming to optimize ML workflows and ensure robust model management.
MLOps Fundamentals Interview Questions Answers - For Intermediate
1. What is MLOps and why is it important?
MLOps combines machine learning (ML) and DevOps practices to streamline the lifecycle of ML models, ensuring collaboration between data scientists and operations teams, reducing time-to-market, and improving model reliability.
2. What are the key phases of the MLOps lifecycle?
The key phases include data engineering, model development, model deployment, continuous integration/continuous delivery (CI/CD), monitoring, and model retraining.
3. How does version control work in MLOps?
Version control in MLOps tracks changes to code, datasets, and models, enabling reproducibility, collaboration, and auditability. Tools like Git and DVC (Data Version Control) are commonly used.
4. What is Continuous Integration (CI) in MLOps?
CI in MLOps involves automatically testing and integrating changes to ML code (like model updates) into a shared repository to ensure model quality and avoid integration issues.
5. What is Continuous Delivery (CD) in MLOps?
The CD focuses on automating the deployment of models into production environments, ensuring that models are consistently updated and deployed in a repeatable and error-free manner.
6. What is model monitoring in MLOps, and why is it crucial?
Model monitoring involves tracking model performance in production environments to detect issues like data drift, concept drift, or reduced accuracy, allowing timely retraining or adjustments.
7. What is the role of a feature store in MLOps?
A feature store is a centralized repository that manages, serves, and reuses ML features across models, ensuring consistency between training and production environments.
8. How does data drift affect ML models in production?
Data drift occurs when the input data distribution changes over time, causing the model’s performance to degrade. Monitoring for drift ensures timely retraining or updating of models.
9. What tools are commonly used for experiment tracking in MLOps?
Tools like MLflow, TensorBoard, and Weights & Biases are commonly used for tracking model experiments, helping in comparing performance metrics and hyperparameters across model versions.
10. What is the significance of CI/CD pipelines in MLOps?
CI/CD pipelines automate the building, testing, and deployment of ML models, ensuring models are integrated and deployed efficiently and consistently, improving productivity and reliability.
11. How do you manage model reproducibility in MLOps?
Reproducibility is ensured by versioning code, data, and models, using containerization (like Docker), and tracking experiments to ensure the same results can be reproduced under identical conditions.
12. How do you handle model retraining in MLOps?
Model retraining is triggered based on monitoring signals like data drift or reduced accuracy. The pipeline is automated to retrain, validate, and redeploy the updated model.
13. What is the difference between batch and real-time model inference in MLOps?
Batch inference processes data in batches, suitable for tasks like periodic predictions, while real-time inference processes data as it arrives, used for tasks requiring instant predictions (e.g., recommendation systems).
14. How do you use Docker in MLOps?
Docker is used to containerize ML models and their dependencies, ensuring consistency between development and production environments, simplifying deployment, and enabling scalability.
15. What are the key benefits of implementing MLOps?
MLOps enhances collaboration between teams, improves model deployment speed, ensures model reliability, enables continuous model improvements, and reduces operational challenges in ML model lifecycle management.
16. What is the purpose of using Kubernetes in MLOps?
Kubernetes helps manage containerized applications, enabling the scaling, deployment, and orchestration of ML models across clusters for efficient, fault-tolerant production environments.
17. What role does automated testing play in MLOps pipelines?
Automated testing in MLOps ensures model code, data pipelines, and model performance meet expected standards by running unit tests, integration tests, and validation checks before deployment.
18. How does model explainability fit into MLOps?
Model explainability tools (e.g., SHAP, LIME) are integrated into MLOps pipelines to provide transparency in model decisions, which is critical for regulatory compliance and trust in AI systems.
19. What is A/B testing in MLOps, and how is it useful?
A/B testing involves comparing two model versions (A and B) in production to evaluate performance differences. It helps in validating model improvements before full deployment.
20. What are the challenges of deploying ML models at scale, and how do MLOps address them?
Scaling ML models involves managing infrastructure, monitoring, retraining, and ensuring consistency across environments. MLOps provides automation, scalability, and reproducibility to handle these challenges effectively.
MLOps Fundamentals Interview Questions Answers - For Advanced
1. How can you implement end-to-end automation in an MLOps pipeline?
End-to-end automation involves integrating CI/CD pipelines for data ingestion, feature engineering, model training, validation, deployment, and monitoring, using tools like Jenkins, Airflow, and Kubernetes to automate each step.
2. What are the best practices for managing model versioning in production environments?
Best practices include using tools like MLflow or DVC for model tracking, tagging models with metadata, maintaining clear audit trails, and ensuring compatibility between model versions using APIs or containerization (e.g., Docker).
3. How do you handle infrastructure scaling for real-time model inference in MLOps?
Infrastructure scaling is managed by leveraging cloud platforms (e.g., AWS Sagemaker, Google AI Platform) and orchestration tools like Kubernetes to auto-scale resources based on traffic, ensuring high availability and low latency.
4. What strategies can be employed to monitor and mitigate model bias in MLOps pipelines?
Strategies include integrating fairness metrics (e.g., disparate impact, equal opportunity), using explainability tools (like SHAP), retraining models on unbiased data subsets, and automating bias detection alerts within the monitoring framework.
5. How can you design an effective feedback loop for model improvement in MLOps?
An effective feedback loop involves capturing real-world performance metrics, integrating user feedback, monitoring model drift, and automating retraining and deployment processes to continuously improve model accuracy and reliability.
6. How do you ensure regulatory compliance in an MLOps framework, particularly for sensitive domains like healthcare or finance?
Compliance is ensured by integrating explainability tools, documenting data lineage, tracking model decisions with auditable logs, enforcing data privacy and implementing model governance frameworks to comply with regulations (e.g., GDPR, HIPAA).
7. What are the challenges of implementing multi-cloud MLOps pipelines, and how can they be addressed?
Multi-cloud challenges include handling data security, ensuring portability, managing differences in cloud services, and preventing vendor lock-in. Solutions include using containerization (e.g., Docker, Kubernetes) and cross-cloud ML tools (e.g., Kubeflow).
8. How can you implement CI/CD pipelines for machine learning models with non-deterministic outputs?
For non-deterministic models, pipelines should focus on statistical testing rather than strict pass/fail criteria. Tolerance ranges for model performance metrics should be set, and validation pipelines should account for variability in model predictions.
9. What are the benefits of using a feature store, and how does it impact the MLOps lifecycle?
A feature store ensures consistency between training and inference, reduces feature engineering duplication, improves feature discovery and reusability, and streamlines the model deployment process, especially in real-time ML systems.
10. How do you manage the challenge of data drift and concept drift in a production MLOps environment?
Data and concept drift can be managed by implementing continuous monitoring systems, comparing current and historical data distributions, triggering automated model retraining pipelines when drifts are detected, and using online learning algorithms.
Course Schedule
Feb, 2025 | Weekdays | Mon-Fri | Enquire Now |
Weekend | Sat-Sun | Enquire Now | |
Mar, 2025 | Weekdays | Mon-Fri | Enquire Now |
Weekend | Sat-Sun | Enquire Now |
Related Courses
Related Articles
Related Interview
Related FAQ's
- Instructor-led Live Online Interactive Training
- Project Based Customized Learning
- Fast Track Training Program
- Self-paced learning
- In one-on-one training, you have the flexibility to choose the days, timings, and duration according to your preferences.
- We create a personalized training calendar based on your chosen schedule.
- Complete Live Online Interactive Training of the Course
- After Training Recorded Videos
- Session-wise Learning Material and notes for lifetime
- Practical & Assignments exercises
- Global Course Completion Certificate
- 24x7 after Training Support
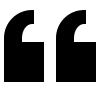